There are many use cases for machine learning in supply chains. The benefits of machine learning and artificial intelligence can be traced back to every part of the supply chain, including procurement, manufacturing, inventory management, warehousing, logistics, and customer service. How to ensure effective supply chain management? This is an open question for many suppliers, distributors, manufacturers, and retailers. In this article, we’ll explore how AI and ML can improve modern supply chain management.
Table of Contents
What Are AI and ML?
Artificial intelligence is a combination of different processes and algorithms. AI can simulate certain aspects of human intelligence, such as self-learning, problem-solving, and responding to a given input. Machine learning and deep learning are subsets of artificial intelligence solutions.
Machine learning falls under the “limited memory” category of AI, where AI solutions can learn and develop themselves over time. Different ML algorithms are used in AI solutions to increase efficiency.
These powerful AI/ML solutions, such as those created by AltaML, are used to address some of the challenges facing the supply chain industry.
Supply Chain & Supply Chain Management
A supply chain is the combination of all the activities required to move a product/service from its inception to the end-user. A supply chain includes people, resources, information, channels, and modes of transportation. All these entities are linked to completing the procurement-to-fulfillment cycle. Reverse logistics also plays a role; consider waste management for fast fashion or recycling. In this regard, it is not just a supply chain, but a circular process.
Supply chain management can be defined as a process that integrates all activities to meet the demand and supply life cycle. The COVID-19 pandemic has had a very negative impact on global supply chains. Organizations that have been focusing on lean supply chain management to optimize costs and meet end-user needs now need to consider risk management and mitigation. High levels of efficiency and visibility in supply chain management thanks to technologies such as AI/ML.
Pain Points in Logistics and Supply Chain Management
Supply chain management is a very complex process. The pandemic has created a lot of uncertainty in global supply chains. This set of challenges includes logistics and transportation issues, increased customer expectations, unexpected demand, lack of visibility, and operational complexity.
Let’s try to summarize these pain points:
- Demand and Supply Planning: Unexpected increases and decreases in demand lead to speculative order placement and lead excess inventory. A proper inventory management system helps organizations keep supply and demand in balance and reduces the “bullwhip effect,” where small fluctuations are amplified when upstream.
- Reactive management: Unplanned events and uncertain notifications mean management is constantly reacting rather than proactively planning ahead. The harmful effects of a lack of scenario planning are evident during a pandemic.
- Supply Network Planning: Lack of upstream and downstream planning in the network can lead to inventory shortages or excesses. It can also lead to deployment issues across networks. Lack of inventory can lead to long wait times and potential loss of customers.
- Security and quality inefficient supply chains make it difficult to deliver products/services on time. Therefore, maintaining proper quality processes and safety becomes a challenge.
- Lack of Information Management: Critical and necessary information is not always available when needed. This results in lost sales and profit margins.
- Scarcity of resources: This is a well-known problem in supply chains. Due to resource shortages, logistics and supply chains cannot function effectively.
- Cost inefficiencies: Financial planning is very important for any supply chain. Organizations must have the financial flexibility and backup plans to sustain disruption and increased costs.
- Technical downtime: Any technical downtime can cause problems in the supply chain process. Therefore, an appropriate backup and failover strategy should be in place to support downtime.
Role of Machine Learning in Supply Chain
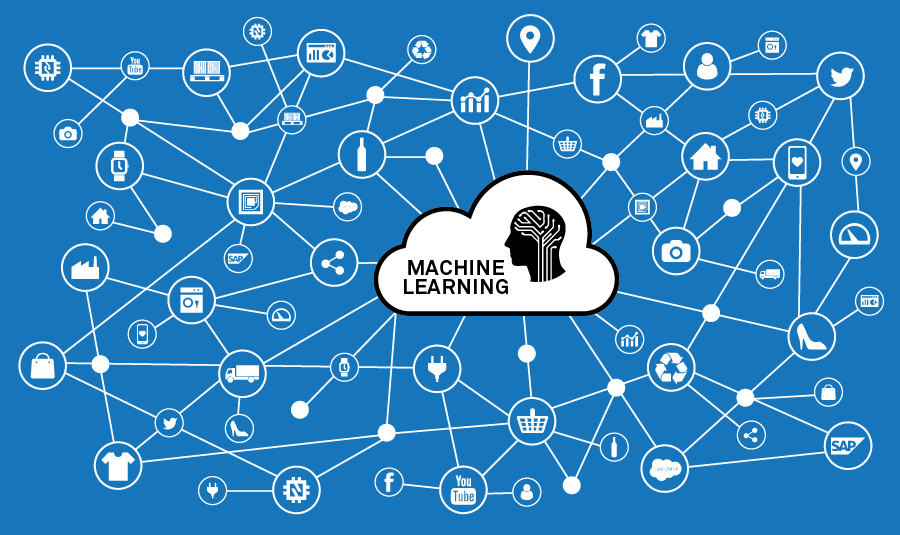
The open question is – how do you make your supply chain less vulnerable to uncertainty? The market dynamics of supply chain management have changed considerably due to factors such as changing workflows and fluctuating demand. The supply chain is no longer a linear deterministic process where workflow is a step-by-step predefined sequence. Instead, the supply chain is now an indeterminate workflow where sequences can be shuffled to optimize the process. Automation is needed to create better supply chain management. (Also read: How can machine learning support supply chains?)
First, integrating AI/ML in the supply chain process can automate a variety of common and repetitive tasks. Applying intelligent ML models can help organizations choose the best option and run their business efficiently. AI solutions can analyze vast amounts of data collected from warehouses, logistics, suppliers, and transportation systems to predict supply and demand and balance the entire ecosystem. The benefits of AI-driven systems can be found at every step of the chain, from inception, procurement, order processing, and inventory to logistics and end-user delivery.
10 Ways Machine Learning Is Revolutionizing Supply Chain Management
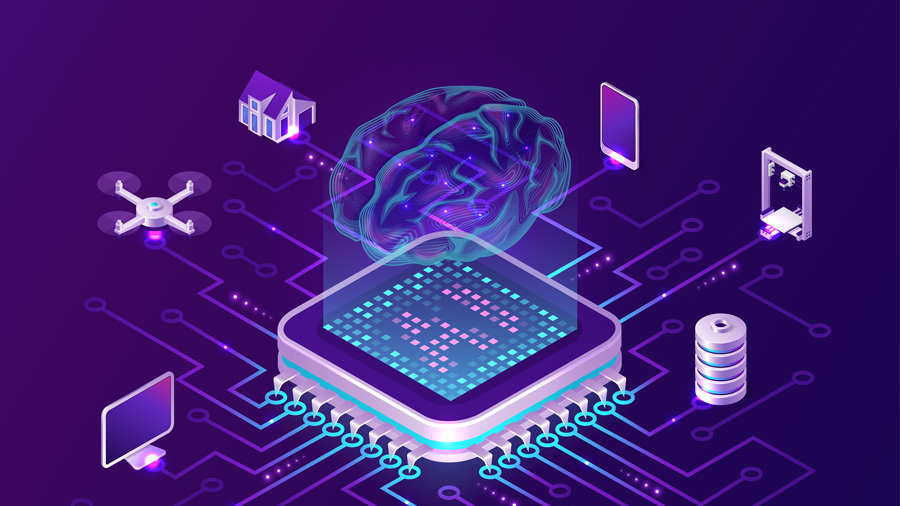
Ten ways machine learning is revolutionizing supply chain management include:
- Machine learning algorithms and the applications that run them can rapidly analyze large, diverse data sets and improve the accuracy of demand forecasts. One of the most challenging aspects of managing a supply chain is predicting future production needs. Existing techniques include baseline statistical analysis techniques (including moving averages) and advanced simulation modeling. Machine learning has proven to be very effective at accounting for factors that existing methods cannot track or quantify over time. The example below shows how demand forecasting can be done using a broad spectrum, and Lennox is now using machine learning.
- Three of the many benefits that machine learning provides in a collaborative supply chain network are lower freight rates, improved supplier delivery performance, and minimized supplier risk. Below is an example of how machine learning can be used today to identify horizontal collaborative synergies between multiple shipper networks.
- Machine learning and its core structures are ideally suited to provide insights for improving supply chain management performance than previous technologies have not been able to provide. Combining the advantages of unsupervised learning, supervised learning, and reinforcement learning, machine learning is proving to be a very effective technique for continuously finding key factors affecting supply chain performance. Each endpoint defined in the taxonomy below is fully derived from algorithm-based logic, which ensures the scalability of the algorithm across the global enterprise.
- Machine learning excels at visual pattern recognition, opening up many potential applications in the physical inspection and maintenance of physical assets across supply chain networks. Machine learning is designed using algorithms that quickly find comparable patterns in multiple datasets, and machine learning has proven to be very effective in automating inbound quality checks across logistics centers, isolating damage and wear and tear in product shipments. Machine learning algorithms in the IBM Watson platform are able to determine shipping containers and . or product damage, categorized by time of damage, and recommend the best corrective action to repair the asset. Watson combines visual and system-based data to track, report, and make recommendations in real-time.
- Gaining greater contextual intelligence by combining machine learning with relevant technologies in supply chain operations can reduce inventory and operational costs and improve response time to customers. The growing adoption of machine learning in logistics control tower operations provides new insights into how to improve all aspects of supply chain management, collaboration, logistics, and warehouse management. The diagram below shows how contextual intelligence gained from machine learning can optimize operations.
- Predicting demand for new products, including the causal factors that will best drive new sales, is one area where machine learning is currently being used with great results. From the pragmatic approach of asking channel partners, and indirect and direct sales teams how many new products they will sell, to using advanced statistical models, the way companies forecast demand for next-generation products varies widely. Machine learning has proven valuable in considering causal factors affecting demand that was previously unknown.
- Companies are extending the life of critical supply chain assets, including machinery, engines, transportation, and warehouse equipment, by discovering new patterns of usage data collected through IoT sensors. Manufacturing leads all other industries in the amount of data generated each year. Machine learning has proven invaluable in analyzing Machine-derived data to determine which causal factors have the greatest impact on machine performance. Additionally, machine learning is leading to more accurate measurements of overall equipment effectiveness (OEE), a key metric on which many manufacturers and supply chain operations rely.
- Improve supplier quality management and compliance by uncovering patterns in supplier quality levels and creating unassisted tracking and tracing data hierarchies for each supplier. On average, more than 80 percent of a typical company’s components are assembled by outside suppliers. The need for supplier quality, compliance, and a track-and-trace hierarchy is critical in regulated industries, including aerospace and defense, food and beverage, and medical products. Machine learning applications are being introduced that can independently define product hierarchies and simplify track and trace reporting, saving typical manufacturers the investment of thousands of man-hours per year in these areas.
- Machine learning improves the accuracy of production planning and factory scheduling by considering multiple constraints and optimizing each constraint. In manufacturers that rely on make-to-order and make-to-stock workflows, machine learning enables it to balance each constraint more efficiently than manually in the past. As a result, manufacturers are using machine learning to reduce supply chain delays for components used in their most customized products.
- Combining machine learning with advanced analytics, IoT sensors and real-time monitoring provide end-to-end visibility across many supply chains for the first time. What many supply chains need today is a new operational platform or architecture based on real-time data, which contains patterns and insights that cannot be seen with the analytical tools used in the past. Machine learning is an important element in future supply chain platforms that will revolutionize every aspect of supply chain management.
Machine Learning Use Cases in Supply Chain Management
Supply chain management is a very complex process that relies heavily on multiple types of data. At every stage of the supply chain, data is collected and processed. Today, artificial intelligence applications, such as those created by AltaML, play a major role in the supply chain industry.
Machine learning is used to process large amounts of input data and train ML, models. As a result, ML models can predict more accurate results and train themselves over a period of time. Here are some interesting ML use cases in supply chains.
- Inventory and warehouse management: Inventory and warehouse management are key use cases for ML implementation. Inventory planning should be very effective to balance demand and supply cycles. ML algorithms can be applied to data collected from various sources such as historical data, seasonal demand, market movements (up and down), and promotions. The findings can be used to improve inventory storage efficiency. Similarly, different ML models are also used to automate warehouse processes.
- Logistics management: Machine learning is used to track the location of goods from pickup to delivery. ML is also used to predict optimized shipping routes, as well as the most efficient modes, optimal lead times, and GHG emissions for the selected mode.
- Production and Quality Management: With the help of ML, the quality of products can be checked and conformed to the required specifications. Therefore, the production line is always well controlled and maintained. Computer vision can be used to facilitate quality control management practices for products on factory production lines, which are important for everything from food to auto parts.
- Predictive Analytics: Predictive analytics is very important for supply and demand management. ML can help predict demand in advance. Therefore, the inventory is always balanced and optimized. Investments can be proactively redeployed within the network based on demand sensing signals.
- Security and Fraud Prevention: Machine learning models can analyze large amounts of data and alert on fraudulent activity. For example, duplicate payments to suppliers can be flagged and potential fraudulent charges reduced. ML can be used to implement anti-fraud processes and strengthen security.
- Delivery Tracking and Customer Service: ML is also used to track the delivery of goods at every stage. External data sources can be used to reduce lead time forecast errors. This has been demonstrated, with an 85% increase in accuracy when packages are delivered from overseas. Therefore, customers are always updated with the latest status. It increases customer satisfaction and controls end-to-end delivery.
How to Make ML Work In Supply Chain Management
There are three important steps to adopting machine learning in supply chain management. they are:
Understand your supply chain structure.
Before applying machine learning to a supply chain, you should evaluate the structure of the entire supply chain:
- Identify the key components of the operation.
- A detailed analysis of the supplier network, including first-tier and second-tier suppliers.
- Identify hidden relationships and interconnecting nodes.
- Quantitatively diagnose the relative vulnerability of supply chains.
- Identify bottlenecks and risk factors in the supply chain.
- Make meaningful comparisons with peers and industry benchmarks.
- Evaluate supply chain security.
- Assess your functional maturity based on process, people, and technology.
Establish transparent business KPIs and calculate ROI.
In order to understand when a machine learning use case in the supply chain is beneficial to your business, you need to go through the discovery phase and calculate the ROI. You need to estimate the total cost of ownership and the profitability you will gain in the short and long term.
Also, it is important to have a detailed plan that defines the goals and requirements needed to achieve the goals. To eliminate inconsistencies, machine learning KPIs must be aligned with business KPIs. In other words, you should define your business problem in ML terms.
Ensuring an effective ML engineering process.
The success of machine learning use cases in supply chains depends heavily on the following:
Build a multifunctional team of professionals with expertise in data science, DevOps, Python, Java, QA, business analytics, and more.
- Start with a business problem statement.
- Establish the right metrics for success.
- Choose the right tech stack.
- Consider your data readiness: focus on data quality and quantity.
- Develop, train, test, and optimize models.
- Deploy and retrain the model.
- Monitor model performance.
Conclusion
The use cases of machine learning in supply chain management are manifold. Here, we list the factors that bring the most value to supply chain professionals. Supply chain management can become a daunting task if you have to manage an extensive network of suppliers, warehouses, and logistics service partners. But technologies like machine learning and artificial intelligence can help you at every stage of supply chain management. ML algorithms will correctly predict demand, improve logistics management, help you reduce paperwork, and automate manual processes. As a result, you gain end-to-end visibility into your supply chain while ensuring it works more efficiently, requires less operating costs, and is less prone to disruption.